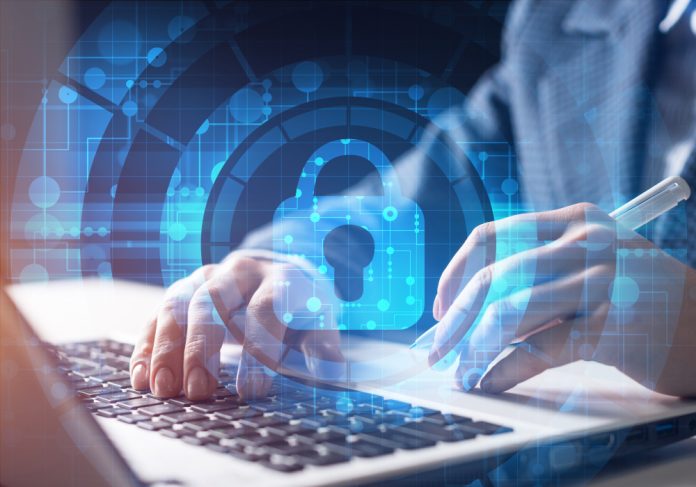
Generative AI (genAI) programs, such as ChatGPT, have captured the imaginations of corporate training professionals who recognize their potential for significantly improving productivity. However, using publicly available AI tools can introduce security risks, and they are notorious for delivering wildly inaccurate information. Moreover, corporate training and other learning and development (L&D) content generally comprises proprietary information that needs to remain private and highly protected.
Some enterprises have responded to the risks by establishing policies that prohibit the business use of genAI—even private applications of the technology using their own internal data. But as adoption grows, organizations that shun genAI for content, such as course materials, certification and assessment guides, and knowledge bases, ultimately may put themselves at a competitive disadvantage.
Instead, companies should start implementing a phased approach to adopting AI for L&D and training content that includes guardrails to protect the integrity, privacy, and safety of these resources.
Take an Enterprise Generative AI Approach
Already, 80 percent of Fortune 500 companies have employees using ChatGPT for work, according to OpenAI. Here’s the catch: That statistic comes from registered ChatGPT consumer accounts associated with corporate e-mail domains. In other words, there is no definitive corporate control over these users.
So the first step in any organization’s use of genAI for training and L&D content should be to adopt an enterprise version that enables companies to work with their own data and content sources in an environment with advanced security and privacy protections. Some of these enterprise-class tools include OpenAI’s ChatGPT Enterprise, Microsoft’s Bing Chat Enterprise, and Google’s Gemini for Google Workspace. Beyond supporting security and privacy, they also enable high degrees of customization.
Increasingly, enterprise content management and learning management system vendors are providing application programming interfaces (APIs) and other integrations to genAI tools that facilitate implementing these technologies. Yet, having the right technology is only part of the equation.
Organizations need to evaluate their content development and governance workflow to identify areas where AI can be utilized and where human review is required. Governance policies should include role-based access control, trackability of content modifications by humans or AI, and proper structuring and tagging of content for downstream AI retrieval. Companies with established design and voice guidelines will have an advantage, as these guidelines can be used to guide AI tools.
Enterprises also need to think about enacting and enforcing corporate policies for how and when generative AI functionality is used, including whether AI tools will only be applied to internal content or also to content from authorized third-party sources. This will be a cross-functional effort that brings together members of the legal, human resources, information technology, and compliance teams in addition to leaders within the training, learning and development, and technical communications teams.
Start with Simple Productivity Gains
People and generative AI tools both get better with training over time. So once a secure environment is available, training and L&D content teams should start with fairly simple uses of their enterprise genAI software to automate more rote functions, recognize early productivity improvements, and gain insights into more strategic applications of the content. Here are three common examples:
- Enforce style guide rules. Paste rules from the organization’s style guide or standard resources, such asThe Chicago Manual of Style and Microsoft Manual Style, and instruct the AI tool to enforce those rules. Then, L&D content teams can submit prompts, such as, “Edit this text to comply with The Chicago Manual of Style rules.”
- Use the company’s established “voice.” Paste text representing an example of the voice applied to training/L&D content and instruct the AI tool to apply that same tone of voice to all future responses. Then, for instance, when a subject matter expert (SME) contributes content, submit a prompt such as, “Apply the exact L&D style and tone of voice to this content.”
- Summarize text or video content. Paste a link to content, such as an instruction guide or training video, into the AI tool, and instruct it to summarize the content. The summary then can be edited and, for example, added to a training resource portal with a link to the full guide or video.
Initially when using the generative AI tool, there will be multiple iterations of prompts as training and L&D content teams work to produce the ones that get the most accurate and consistent responses. So it can be useful to set up a train-the-trainer program where a small group of experts pilot the genAI tool for various functions and then educate colleagues on established processes and prompts as they evolve.
Plan for More Strategic Uses
Many training and L&D teams need to produce more content faster, so even using genAI to automate rote processes can translate into tangible productivity gains. Yet, far more potentially game-changing applications of genAI are still on the horizon.
Picture using genAI to match the right training content to a particular person based on their role, success with earlier training programs and content, and even experiences with the support team. Imagine AI improving the extraction of knowledge from SMEs and other experts into the enterprise knowledge base. Or consider having an AI tool analyze how much of the organization’s training content is for expert versus novice users—or where there are notable gaps—in order to inform and refine the L&D team’s content development strategy.
Today, most enterprise content is not structured to support such future applications of genAI. Current content silos across training, support, product, and other business groups need to be connected. Large blocks of content need to be componentized, and metadata tags need to be applied to facilitate search and analysis.
As training and L&D teams build expertise in using enterprise genAI tools, organizations also should begin making foundational changes to the way their content is stored and managed to fully capitalize on the potential of generative AI tomorrow.